The Ethics AI Revolution: E&C Cybersecurity Considerations ๐ค๐พ
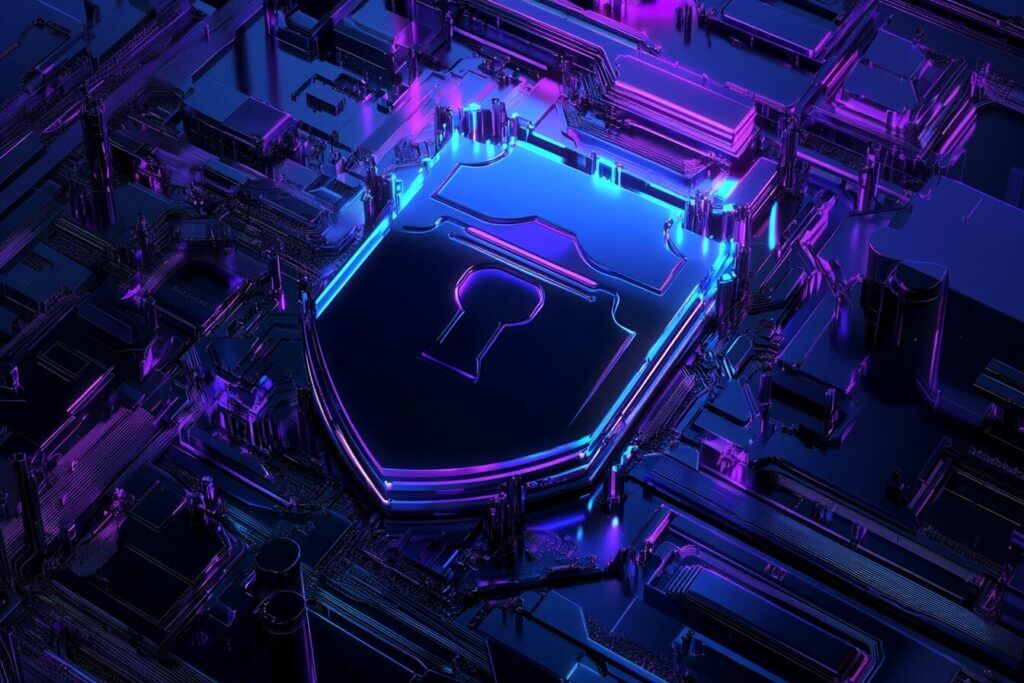
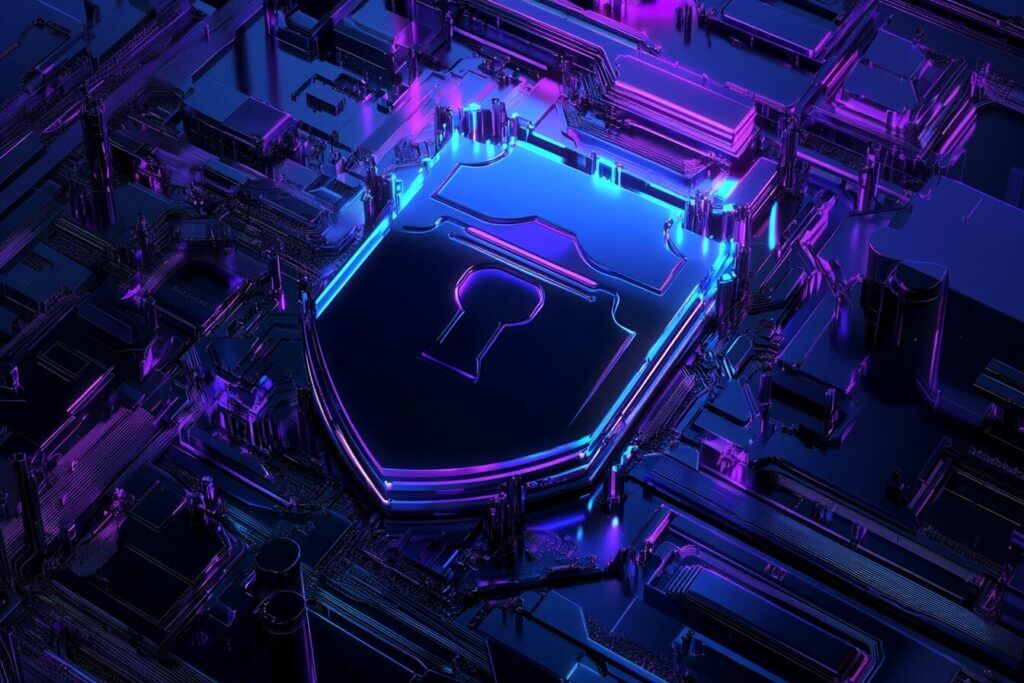
Full Episode Available
WATCH ON-DEMANDAs healthcare organizations navigate the rapid evolution of artificial intelligence technologies, leaders face the dual challenge of leveraging AI’s transformative potential while safeguarding against increasingly sophisticated cyber threats. This webinar brings together frontline experts from healthcare technology, compliance, and AI consulting to provide practical insights on implementing AI solutions safely and effectively. Whether you’re evaluating AI vendors, building governance frameworks, or working to protect patient data in an AI-enabled environment, this session offers actionable strategies for balancing innovation with security.
This comprehensive session brought together healthcare technology leaders, compliance experts, and AI consultants to explore the intersection of artificial intelligence, healthcare operations, and cybersecurity. The discussion covered the full spectrum of AI implementation in healthcare settings, from basic rules-based systems to advanced generative AI applications, while addressing critical considerations around security, privacy, and ethical deployment. Speakers emphasized the importance of balanced adoption strategies that maximize AI’s benefits while maintaining robust security and compliance frameworks.
Meet The Ethics Experts:
- Gerry Blass, President & CEO, ComplyAssistant
- Jack Hueter, CEO & Owner, Digital Healthcare Consulting
- Richard Kerr, Administrator, Clinical Applications, Lehigh Valley Health Network
- Sabina Zafar, Founder & CEO at AI Cloud Consulting Group, Managing Partner at Valenta
- Martin von Grossman, Senior Consultant, ComplyAssistant
- Giovanni Gallo, Co-CEO, Ethico
Understanding AI’s Evolution in Healthcare
- The progression of AI from basic rules-based systems to advanced generative AI represents different levels of complexity and risk. Healthcare organizations must understand where various AI solutions fall on this spectrum to implement appropriate governance and security measures.
- Current applications range from clinical decision support and imaging analysis to operational efficiency and revenue cycle management. Organizations should recognize that different AI technologies require different levels of validation.
- ย This understanding should inform everything from vendor selection to implementation strategies and ongoing monitoring protocols.
Governance and Oversight Frameworks
- Successful AI implementation requires a multi-disciplinary steering committee including clinical leadership, IT, compliance, ethics, and operational stakeholders. Organizations should establish clear policies for AI adoption, regular assessment protocols, and continuous monitoring of AI system performance.
- This includes addressing data drift, model bias, and maintaining transparency in AI-driven decision-making. The governance framework should include specific processes for evaluating new AI implementations, monitoring existing systems, and ensuring appropriate clinical oversight of AI-assisted decisions.
- Regular reviews should assess both technical performance and clinical outcomes, with clear escalation paths for addressing concerns or unexpected results.
Third-Party Risk Management
- Healthcare organizations must carefully evaluate AI vendors and third-party solutions, particularly regarding data handling, privacy, and security practices. This includes reviewing business associate agreements, understanding data flows, and ensuring appropriate security controls are in place.
- Regular assessments of third-party AI systems should be conducted to maintain compliance and security standards. Organizations should develop specific criteria for evaluating AI vendors, including their data sources, training methodologies, and ability to explain their models’ decisions.
- Vendor contracts should include specific provisions for model updates, data handling, and performance monitoring, with clear accountability for maintaining system accuracy and reliability.
Security and Privacy Considerations
- AI implementations require robust security measures including encryption, access controls, and regular vulnerability assessments. Organizations must address both traditional cybersecurity concerns and AI-specific risks such as model manipulation and data poisoning.
- Privacy considerations should include consent mechanisms for AI use in patient care and clear protocols for data handling. Security frameworks must be updated to account for new AI-specific vulnerabilities while maintaining traditional security controls.
- Organizations should implement specific monitoring for AI system access and usage, with particular attention to data flows and potential exposure points.
Clinical Implementation Strategy
- Healthcare providers should focus on practical use cases where AI can demonstrate clear value, such as imaging analysis, clinical documentation, and decision support tools. Implementation should be gradual and targeted, with careful attention to clinician training, workflow integration, and ongoing performance monitoring.
- Success metrics should be clearly defined before implementation, with regular assessment of both clinical outcomes and operational efficiency.
- Organizations should start with well-defined, bounded use cases where AI can augment existing processes rather than attempting wholesale transformation.
Cybersecurity Threat Landscape
- AI is being used both defensively and offensively in cybersecurity. Organizations must be aware of AI-enabled threats such as sophisticated phishing attacks, deepfakes, and automated malware, while also leveraging AI for threat detection and response.
- Regular training and awareness programs should address these evolving threats. Security teams need to understand how AI can be used to both enhance and compromise security, developing specific protocols for detecting and responding to AI-enabled threats.
- This includes understanding new attack vectors such as model poisoning and adversarial attacks specific to AI systems.
Compliance and Regulatory Considerations
- Organizations must stay current with evolving regulatory frameworks, including HIPAA updates and new AI-specific regulations. Compliance programs should address consent requirements, documentation standards, and audit trails for AI systems.
- Regular assessments should verify compliance with both existing and emerging regulatory requirements. This includes developing specific documentation requirements for AI-assisted decisions, maintaining clear audit trails of AI system usage, and ensuring appropriate disclosure to patients.
- Organizations should also prepare for upcoming regulatory changes, including potential new requirements for AI transparency and accountability.
ย Change Management and Training
- Successful AI implementation requires comprehensive change management strategies and ongoing training programs. Staff must understand both the capabilities and limitations of AI systems, including potential biases and the importance of human oversight in decision-making processes.
- Training should be role-specific, with clinical staff receiving different training than administrative staff. Organizations should develop clear protocols for when and how to override AI recommendations, ensuring staff understand their role in maintaining quality and safety.
- Regular refresher training should address new capabilities and lessons learned from system use.
Data Quality and Interoperability
- The effectiveness of AI systems depends heavily on data quality and accessibility. Organizations should focus on data standardization, reducing bias in training data, and ensuring appropriate data sharing frameworks are in place while maintaining privacy and security standards.
- This includes developing specific protocols for data validation, regular data quality assessments, and processes for identifying and addressing potential bias in training data.
- Organizations should also work to ensure their data infrastructure supports both current and future AI applications while maintaining appropriate security controls.
Future Considerations
- Healthcare organizations must prepare for increasing AI adoption while maintaining focus on responsible implementation. This includes addressing ethical considerations, ensuring equitable access to AI-enabled care, and maintaining appropriate human oversight of AI systems.
- Organizations should develop roadmaps for future AI adoption that balance innovation with risk management, including specific criteria for evaluating new technologies and use cases.
- Planning should include consideration of workforce development, infrastructure needs, and evolving patient expectations around AI-enabled care.
Conclusion
The integration of AI in healthcare presents both significant opportunities and substantial challenges. Success requires a balanced approach that embraces innovation while maintaining robust security, privacy, and ethical standards. Organizations must develop comprehensive frameworks for AI governance while staying adaptable to evolving technologies and regulatory requirements. The focus should remain on using AI to augment and enhance healthcare delivery while carefully managing associated risks and ensuring patient safety and privacy remain paramount.