AI Ethics 2.0: A Deep Dive Into Ethical Tech Integration
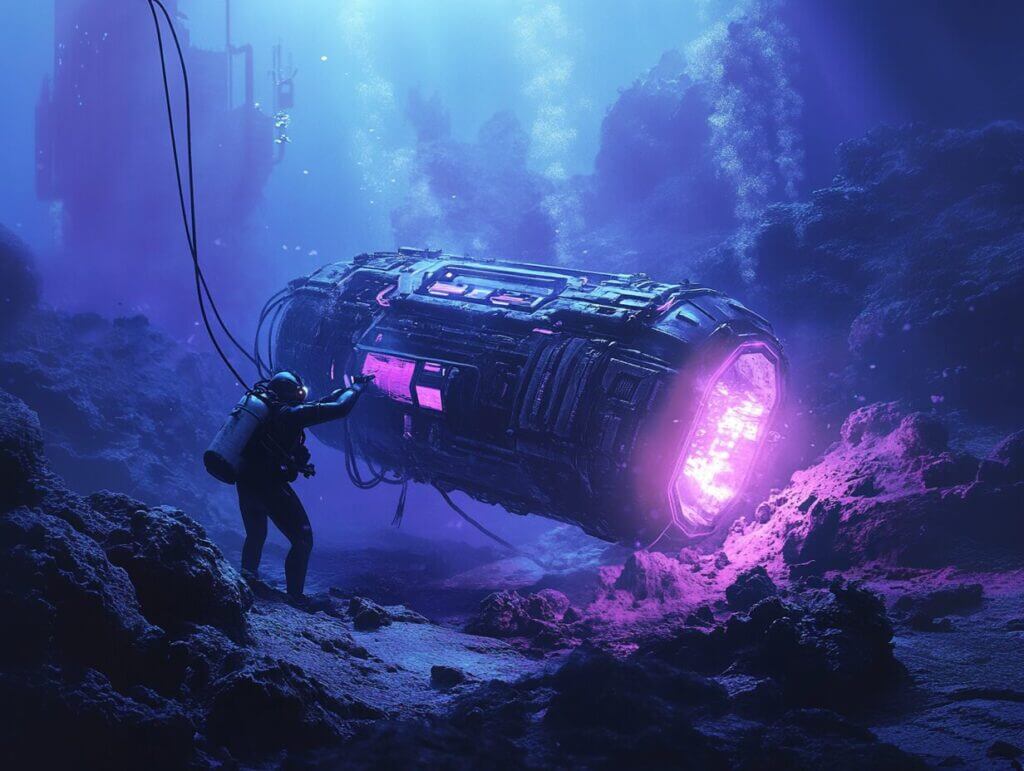
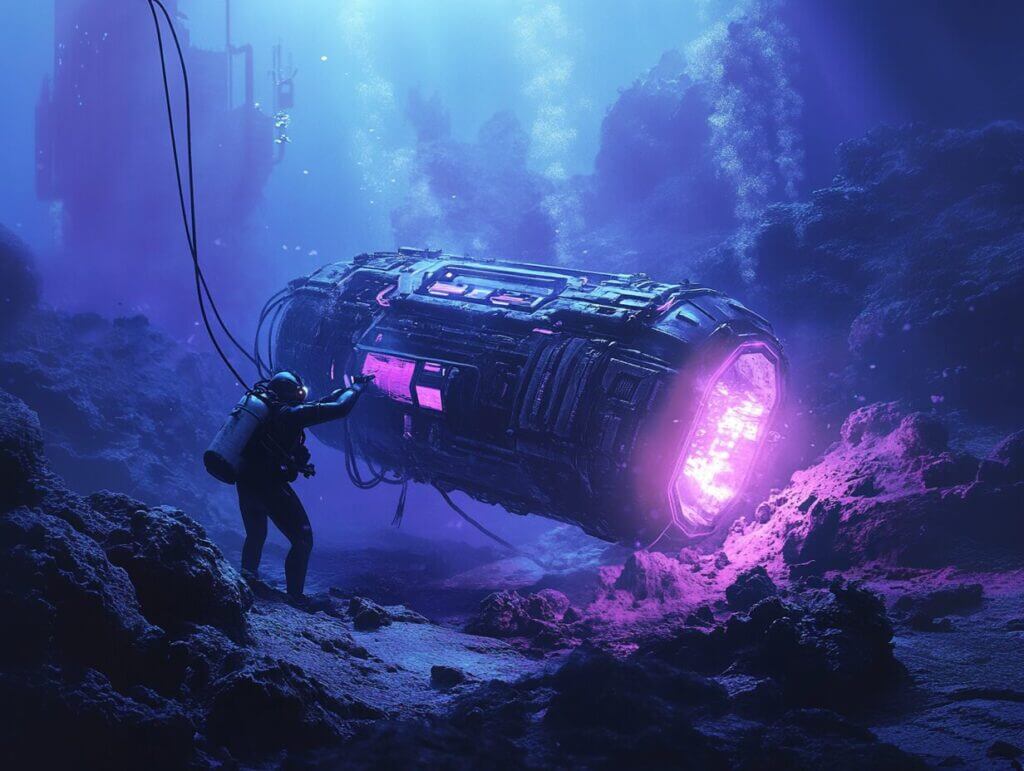
Full Episode Available
WATCH ON-DEMANDArtificial intelligence isn’t just coming to your compliance program – it’s already here, whether you’re ready or not. From AI note-takers automatically transcribing sensitive meetings to employees experimenting with ChatGPT for work tasks, compliance officers are facing a new frontier of risks and opportunities. But here’s the good news: you don’t need to be a technical expert to effectively manage AI in your organization. This discussion cuts through the hype and fear to deliver practical, actionable strategies for compliance professionals wrestling with AI implementation.
This webinar examined the evolving landscape of AI implementation within compliance programs, addressing key challenges and opportunities faced by compliance professionals. The discussion focused on practical approaches to AI risk assessment, data privacy protection, and access control management, while emphasizing the importance of cross-functional collaboration between compliance, information security, and other stakeholders. Speakers highlighted the need for balanced implementation strategies that leverage AI’s benefits while maintaining appropriate controls and oversight. The session provided concrete guidance on policy development, training approaches, and risk mitigation strategies, particularly in light of recent Department of Justice guidance on corporate compliance programs.
Meet The Ethics Experts:
- Diana Kelley, Chief Information Security Officer, Protect AI
- Kristy Grant-Hart, Founder & CEO, Spark Compliance Consulting
- Giovanni Gallo, Co-CEO, Ethico
AI Risk Assessment Framework
- The Department of Justice’s updated evaluation of corporate compliance programs necessitates a three-pronged approach to AI risk assessment: evaluating AI tools within compliance programs, assessing employee use of AI tools, and examining AI products being developed for market release.
- Organizations must consider privacy implications, data security, and potential misuse scenarios when implementing AI solutions. The assessment should incorporate both technical and operational risks, including the potential for data breaches and unauthorized access.
- Regular reviews and updates of risk assessments are crucial as AI capabilities and use cases evolve. Compliance officers should focus on developing clear metrics and monitoring mechanisms for AI-related risks.
Data Privacy and AI Note-Taking
- AI-powered note-taking tools present significant privacy and confidentiality risks, particularly regarding the storage and distribution of sensitive information.
- Organizations must implement strict controls over transcript sharing and establish clear protocols for managing access to AI-generated meeting records. The accuracy of AI transcription needs to be verified, especially for discussions involving sensitive topics or complex terminology.
- Compliance teams should develop specific guidelines for the use of AI note-taking tools in different meeting contexts. Storage and retention policies must align with broader data governance frameworks.
Access Control Management
- Role-based access control for AI systems requires careful consideration of data accessibility and user permissions. Organizations should implement granular controls to prevent unauthorized access to sensitive information through AI tools.
- A critical emerging threat is the “confused pilot attack,” where users manipulate AI assistants like GitHub Copilot or similar internal tools to access information beyond their authorization level.
- Regular audits of AI system access patterns and user behaviors should be conducted. Implementation of proper data classification and tagging systems is crucial for maintaining appropriate access controls.
Security and Privacy Considerations
- AI implementations require robust security measures including encryption, access controls, and regular vulnerability assessments. Organizations must address both traditional cybersecurity concerns and AI-specific risks such as model manipulation and data poisoning.
- Privacy considerations should include consent mechanisms for AI use in patient care and clear protocols for data handling. Security frameworks must be updated to account for new AI-specific vulnerabilities while maintaining traditional security controls.
- Organizations should implement specific monitoring for AI system access and usage, with particular attention to data flows and potential exposure points.
Training and Communication Strategy
- Healthcare providers should focus on practical use cases where AI can demonstrate clear value, such as imaging analysis, clinical documentation, and decision support tools. Implementation should be gradual and targeted, with careful attention to clinician training, workflow integration, and ongoing performance monitoring.
- Success metrics should be clearly defined before implementation, with regular assessment of both clinical outcomes and operational efficiency.
- Organizations should start with well-defined, bounded use cases where AI can augment existing processes rather than attempting wholesale transformation.
Cybersecurity Threat Landscape
- Employee training on AI should be tailored to specific roles and responsibilities while maintaining a baseline understanding across the organization. Training programs should focus on practical applications and risk awareness rather than technical details.
- Training effectiveness should be measured and programs adjusted based on feedback and incident patterns. Organizations should implement scenario-based training modules that simulate real-world AI usage situations and potential ethical dilemmas.
- Additionally, companies should establish mentorship programs where technically proficient employees can guide others in responsible AI use, creating a culture of continuous learning and awareness.
Cross-functional Collaboration
- Successful AI implementation requires effective collaboration between compliance, information security, legal, and business units. Clear delineation of responsibilities and accountability is essential for managing AI-related risks.
- Regular coordination meetings and shared governance structures help ensure comprehensive risk management. Teams should develop joint metrics for measuring AI program effectiveness. Cross-functional input should be incorporated into policy development and risk assessment processes.
- Organizations should establish AI steering committees with representatives from all relevant departments to ensure aligned decision-making. Additionally, regular cross-functional workshops should be conducted to share insights, challenges, and best practices across departments, fostering a more integrated approach to AI governance.
Change Management and Training
- Successful AI implementation requires comprehensive change management strategies and ongoing training programs. Staff must understand both the capabilities and limitations of AI systems, including potential biases and the importance of human oversight in decision-making processes.
- Training should be role-specific, with clinical staff receiving different training than administrative staff. Organizations should develop clear protocols for when and how to override AI recommendations, ensuring staff understand their role in maintaining quality and safety.
- Regular refresher training should address new capabilities and lessons learned from system use.
Policy Development Approach
- AI policies should be principles-based rather than prescriptive, focusing on ethical use, transparency, and risk management. Policies must address both general AI use and specific application scenarios.
- Organizations should maintain flexibility in policy frameworks while ensuring core principles remain consistent. Integration with existing compliance frameworks is crucial for effective implementation.
- Policy development should include input from end-users to ensure practical applicability and adoption. Additionally, organizations should establish clear mechanisms for policy exception handling and rapid updates in response to emerging AI capabilities and risks.
AI Supply Chain Management
- Organizations must carefully evaluate AI vendors and understand the complete supply chain of AI solutions. Contracts should clearly specify data usage rights and privacy protections.
- Regular assessments of third-party AI providers should be conducted to ensure ongoing compliance. Organizations should maintain visibility into AI model training data sources and methods.
- Companies should implement continuous monitoring of AI vendor performance and compliance with contractual obligations. Additionally, organizations should develop contingency plans for vendor transitions or failures, including data portability requirements and model retraining procedures.
Documentation and Auditability
- Proper documentation of AI-related decisions and processes is crucial for maintaining accountability and demonstrating compliance. Organizations should establish clear audit trails for AI system usage and modifications.
- Documentation requirements should be clearly communicated to all stakeholders. Records should be maintained in a format that facilitates external audits and regulatory reviews.
- Organizations should implement automated documentation systems that capture AI decision-making processes and data lineage. Additionally, companies should establish clear procedures for documenting and investigating cases where AI systems produce unexpected or potentially harmful outputs.
Change Management and Cultural Integration
- Organizations must manage the cultural shift associated with AI adoption through clear communication and change management strategies. Employee concerns about AI implementation should be addressed proactively and transparently.
- Regular feedback channels should be maintained to identify and address implementation challenges. Change management programs should focus on building trust in AI systems while maintaining appropriate skepticism.
- Companies should develop internal AI champions programs to facilitate cultural transformation and knowledge sharing. Additionally, regular surveys and focus groups should be conducted to assess employee attitudes and concerns regarding AI implementation.
Regulatory Compliance and Monitoring
- Compliance programs must stay current with evolving regulatory requirements related to AI implementation. Regular monitoring of regulatory changes and updates to compliance frameworks is essential.
- Organizations should maintain flexibility in their compliance programs to adapt to new requirements. Clear protocols for reporting AI-related incidents should be established.
- Organizations should participate in industry groups and regulatory forums to stay informed of emerging compliance requirements and best practices. Additionally, companies should establish dedicated teams or roles responsible for monitoring and interpreting AI-specific regulations across relevant jurisdictions.
Conclusion
The integration of AI into compliance programs represents both significant opportunities and challenges for organizations. Success requires a balanced approach that combines technical controls, clear policies, and effective training while maintaining flexibility to adapt to evolving technologies and regulations. By focusing on practical implementation strategies and maintaining strong cross-functional collaboration, organizations can effectively leverage AI while managing associated risks and maintaining regulatory compliance.